Data Scientist vs. Data Operations Manager
Data Scientist vs Data Operations Manager: A Comprehensive Comparison
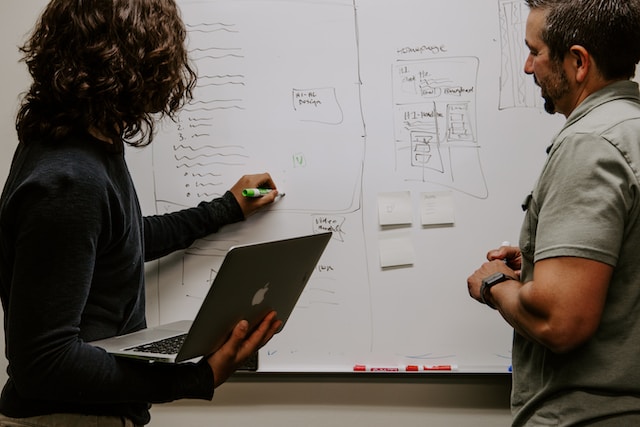
Table of contents
Data Science and Data Operations Management are two of the most sought-after career paths in the field of Artificial Intelligence (AI), Machine Learning (ML), and Big Data. While both these roles are related to managing and analyzing data, they have distinct differences in terms of their job responsibilities, required skills, educational backgrounds, tools and software used, common industries, outlooks, and practical tips for getting started in these careers. This article aims to provide a detailed comparison between Data Scientist and Data Operations Manager roles to help aspiring professionals make informed career choices.
Definitions
A Data Scientist is a professional who uses statistical and mathematical techniques to analyze and interpret complex data sets to derive insights that can help organizations make informed business decisions. They use various programming languages and tools to develop predictive models, build algorithms, and create data visualizations that can help organizations understand their customers, products, and markets better. Data Scientists work with large volumes of data and use advanced Machine Learning algorithms to identify patterns, trends, and insights that can be used to improve business outcomes.
On the other hand, a Data Operations Manager is responsible for managing the entire data infrastructure of an organization. They ensure that data is collected, stored, processed, and analyzed in a secure and efficient manner. Data Operations Managers work with cross-functional teams to develop and implement Data management policies, procedures, and standards that ensure the accuracy, completeness, and integrity of data. They also oversee the deployment and maintenance of data management systems and tools such as databases, data warehouses, and data lakes.
Responsibilities
Data Scientists and Data Operations Managers have different job responsibilities. Some of the key responsibilities of each role are as follows:
Data Scientist
- Collect, clean, and preprocess data from various sources
- Develop and implement machine learning algorithms to analyze data
- Create predictive models to forecast business outcomes
- Build data visualizations and dashboards to communicate insights to stakeholders
- Collaborate with cross-functional teams to identify business problems that can be solved using Data analysis
- Continuously monitor and evaluate the performance of machine learning models and adjust them accordingly
- Stay up-to-date with the latest trends and developments in the field of data science
Data Operations Manager
- Design and implement data management policies, procedures, and standards
- Ensure the accuracy, completeness, and integrity of data
- Develop and maintain data management systems and tools
- Monitor and optimize data processing and storage systems
- Troubleshoot and resolve data-related issues
- Ensure compliance with data Privacy and security regulations
- Collaborate with cross-functional teams to identify data-related business problems and opportunities
Required Skills
Data Scientists and Data Operations Managers require different sets of skills to perform their job responsibilities effectively. Some of the key skills required for each role are as follows:
Data Scientist
- Proficiency in programming languages such as Python, R, and SQL
- Knowledge of machine learning algorithms and Statistical modeling techniques
- Familiarity with data visualization tools such as Tableau, Power BI, and D3.js
- Strong analytical and problem-solving skills
- Excellent communication and presentation skills
- Ability to work in a team environment
- Curiosity and a desire to learn new things
Data Operations Manager
- Proficiency in database management systems such as MySQL, Oracle, and MongoDB
- Knowledge of Data Warehousing and ETL (Extract, Transform, Load) processes
- Familiarity with data management tools such as Apache Hadoop, Spark, and Kafka
- Strong project management and organizational skills
- Excellent communication and interpersonal skills
- Ability to work in a fast-paced and dynamic environment
- Attention to detail and a focus on quality
Educational Backgrounds
Data Scientists and Data Operations Managers typically have different educational backgrounds. Some of the common educational qualifications for each role are as follows:
Data Scientist
- Bachelor's or Master's degree in Computer Science, Mathematics, Statistics, or a related field
- Knowledge of machine learning and statistical modeling techniques
- Familiarity with programming languages such as Python, R, and SQL
- Experience with data visualization tools such as Tableau, Power BI, and D3.js
Data Operations Manager
- Bachelor's or Master's degree in Computer Science, Information Systems, or a related field
- Knowledge of database management systems and data warehousing
- Familiarity with data management tools such as Apache Hadoop, Spark, and Kafka
- Experience with project management and organizational skills
Tools and Software Used
Data Scientists and Data Operations Managers use different tools and software to perform their job responsibilities. Some of the common tools and software used for each role are as follows:
Data Scientist
- Programming languages such as Python, R, and SQL
- Machine learning libraries such as Scikit-learn, TensorFlow, and Keras
- Data visualization tools such as Tableau, Power BI, and D3.js
- Cloud computing platforms such as AWS, Azure, and Google Cloud Platform
Data Operations Manager
- Database management systems such as MySQL, Oracle, and MongoDB
- Data warehousing tools such as Amazon Redshift, Snowflake, and Google BigQuery
- Data management tools such as Apache Hadoop, Spark, and Kafka
- Cloud computing platforms such as AWS, Azure, and Google Cloud Platform
Common Industries
Data Scientists and Data Operations Managers work in different industries and sectors. Some of the common industries for each role are as follows:
Data Scientist
- Technology
- Finance
- Healthcare
- Retail
- Marketing
Data Operations Manager
- Technology
- Finance
- Healthcare
- Retail
- Manufacturing
Outlooks
Data Science and Data Operations Management are two of the fastest-growing and most lucrative career paths in the field of AI, ML, and Big Data. According to Glassdoor, the average salary for a Data Scientist in the United States is $113,309 per year, while the average salary for a Data Operations Manager is $100,000 per year. Both these roles are expected to see significant growth in the coming years, with the demand for skilled professionals in these fields expected to increase exponentially.
Practical Tips for Getting Started
If you are interested in pursuing a career in Data Science or Data Operations Management, here are some practical tips to help you get started:
Data Scientist
- Learn programming languages such as Python, R, and SQL
- Familiarize yourself with machine learning algorithms and statistical modeling techniques
- Gain experience with data visualization tools such as Tableau, Power BI, and D3.js
- Build a portfolio of projects that demonstrate your skills and expertise
- Consider obtaining a certification in Data Science from a reputable institution
Data Operations Manager
- Learn database management systems such as MySQL, Oracle, and MongoDB
- Familiarize yourself with data warehousing and ETL processes
- Gain experience with data management tools such as Apache Hadoop, Spark, and Kafka
- Build a portfolio of projects that demonstrate your skills and expertise
- Consider obtaining a certification in Data Operations Management from a reputable institution
Conclusion
In conclusion, Data Science and Data Operations Management are two distinct career paths in the field of AI, ML, and Big Data. While both these roles involve managing and analyzing data, they have different job responsibilities, required skills, educational backgrounds, tools and software used, common industries, outlooks, and practical tips for getting started. By understanding the differences between these roles, aspiring professionals can make informed career choices and build successful careers in these exciting and rapidly growing fields.
Founding AI Engineer, Agents
@ Occam AI | New York
Full Time Senior-level / Expert USD 100K - 180KAI Engineer Intern, Agents
@ Occam AI | US
Internship Entry-level / Junior USD 60K - 96KAI Research Scientist
@ Vara | Berlin, Germany and Remote
Full Time Senior-level / Expert EUR 70K - 90KData Architect
@ University of Texas at Austin | Austin, TX
Full Time Mid-level / Intermediate USD 120K - 138KData ETL Engineer
@ University of Texas at Austin | Austin, TX
Full Time Mid-level / Intermediate USD 110K - 125KLead GNSS Data Scientist
@ Lurra Systems | Melbourne
Full Time Part Time Mid-level / Intermediate USD 70K - 120K