Research Engineer vs. Data Quality Analyst
Research Engineer vs Data Quality Analyst: A Comprehensive Comparison
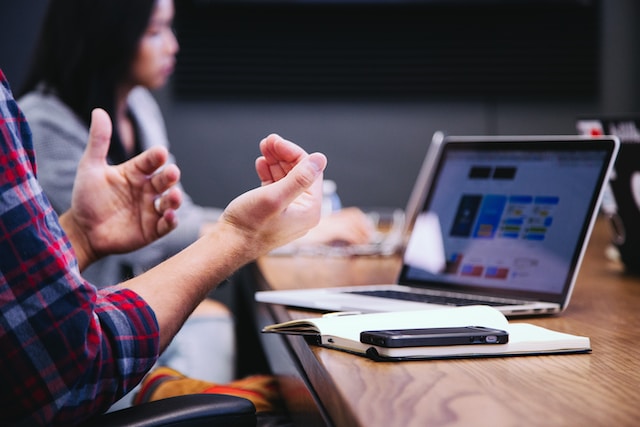
Table of contents
The fields of artificial intelligence, machine learning, and Big Data are rapidly growing, and as a result, so are the career opportunities in these spaces. Two such career paths that are becoming increasingly popular are Research Engineer and Data Quality Analyst. In this article, we will compare and contrast these two roles in terms of their definitions, responsibilities, required skills, educational backgrounds, tools and software used, common industries, outlooks, and practical tips for getting started in these careers.
Definitions
A Research Engineer is a professional who is responsible for designing, developing, and testing new technologies and systems in the field of artificial intelligence and Machine Learning. They work on projects that involve developing algorithms, models, and software that can be used to solve complex problems in various industries.
On the other hand, a Data quality Analyst is responsible for ensuring that data is accurate, complete, and consistent across an organization. They work to identify and correct errors in data and ensure that it is of high quality and can be used effectively for analysis and decision-making.
Responsibilities
The responsibilities of a Research Engineer can vary depending on the industry and company they work for. However, some common tasks include:
- Conducting research on new technologies and techniques in artificial intelligence and machine learning
- Designing and developing algorithms, models, and software that can be used to solve complex problems
- Testing and evaluating the performance of algorithms and models
- Collaborating with other professionals, such as data scientists and software engineers, to develop and implement solutions
- Staying up-to-date with the latest developments in the field and integrating them into projects
The responsibilities of a Data Quality Analyst can also vary depending on the industry and company they work for. However, some common tasks include:
- Identifying and correcting errors in data
- Developing and implementing data quality standards and procedures
- Conducting data audits to ensure data accuracy and completeness
- Collaborating with other professionals, such as data scientists and data analysts, to ensure data is of high quality and can be used effectively for analysis and decision-making
- Staying up-to-date with the latest developments in data quality and integrating them into projects
Required Skills
To be successful as a Research Engineer, one needs to have a strong foundation in mathematics, statistics, and Computer Science. Some other skills that are required include:
- Proficiency in programming languages such as Python, Java, and C++
- Knowledge of machine learning algorithms and techniques
- Experience with software development and testing
- Strong problem-solving and analytical skills
- Good communication and collaboration skills
To be successful as a Data Quality Analyst, one needs to have a strong foundation in Data management, analysis, and quality assurance. Some other skills that are required include:
- Proficiency in SQL and other Data analysis tools
- Knowledge of data quality standards and procedures
- Experience with data auditing and validation
- Strong problem-solving and analytical skills
- Good communication and collaboration skills
Educational Background
To become a Research Engineer, one typically needs a bachelor's or master's degree in computer science, Mathematics, or a related field. Some employers may also require a Ph.D. in a related field.
To become a Data Quality Analyst, one typically needs a bachelor's degree in computer science, information technology, or a related field. Some employers may also require a master's degree in a related field.
Tools and Software Used
Research Engineers typically use a variety of tools and software, including:
- Programming languages such as Python, Java, and C++
- Machine learning libraries such as TensorFlow and PyTorch
- Software development tools such as Git and JIRA
- Cloud computing platforms such as AWS and Google Cloud Platform
Data Quality Analysts typically use a variety of tools and software, including:
- SQL and other data analysis tools
- Data quality software such as Talend and Informatica
- Data visualization tools such as Tableau and Power BI
- Collaboration tools such as Slack and Asana
Common Industries
Research Engineers are in demand in a variety of industries, including:
- Healthcare
- Finance
- Retail
- Manufacturing
- Technology
Data Quality Analysts are in demand in a variety of industries, including:
- Healthcare
- Finance
- Retail
- Manufacturing
- Technology
Outlooks
The outlook for Research Engineers and Data Quality Analysts is positive. According to the Bureau of Labor Statistics, employment of computer and information research scientists, which includes Research Engineers, is projected to grow 15 percent from 2019 to 2029, much faster than the average for all occupations. Similarly, employment of computer and information systems managers, which includes Data Quality Analysts, is projected to grow 10 percent from 2019 to 2029, much faster than the average for all occupations.
Practical Tips for Getting Started
To get started as a Research Engineer, one can:
- Build a strong foundation in mathematics, statistics, and computer science
- Learn programming languages such as Python, Java, and C++
- Gain experience with machine learning libraries such as TensorFlow and PyTorch
- Participate in online communities such as Kaggle and GitHub
- Pursue internships or entry-level positions in the field
To get started as a Data Quality Analyst, one can:
- Build a strong foundation in data management, analysis, and quality assurance
- Learn SQL and other data analysis tools
- Gain experience with data quality software such as Talend and Informatica
- Participate in online communities such as Data Science Central and LinkedIn
- Pursue internships or entry-level positions in the field
Conclusion
In conclusion, Research Engineer and Data Quality Analyst are two career paths in the AI/ML and big data space that require different skill sets and educational backgrounds. While Research Engineers are responsible for designing and developing new technologies and systems, Data Quality Analysts are responsible for ensuring that data is accurate and of high quality. Both career paths are in demand and offer positive outlooks for the future. By building a strong foundation in the required skills and pursuing relevant educational opportunities and experiences, one can successfully launch a career in either of these fields.
AI Research Scientist
@ Vara | Berlin, Germany and Remote
Full Time Senior-level / Expert EUR 70K - 90KData Architect
@ University of Texas at Austin | Austin, TX
Full Time Mid-level / Intermediate USD 120K - 138KData ETL Engineer
@ University of Texas at Austin | Austin, TX
Full Time Mid-level / Intermediate USD 110K - 125KLead GNSS Data Scientist
@ Lurra Systems | Melbourne
Full Time Part Time Mid-level / Intermediate USD 70K - 120KSenior Machine Learning Engineer (MLOps)
@ Promaton | Remote, Europe
Full Time Senior-level / Expert EUR 70K - 110KSenior Cloud Database Administrator (DBA)
@ Cognosante | United States
Full Time Senior-level / Expert USD 113K - 187K