Research Engineer vs. Deep Learning Engineer
Research Engineer vs Deep Learning Engineer: A Comprehensive Comparison
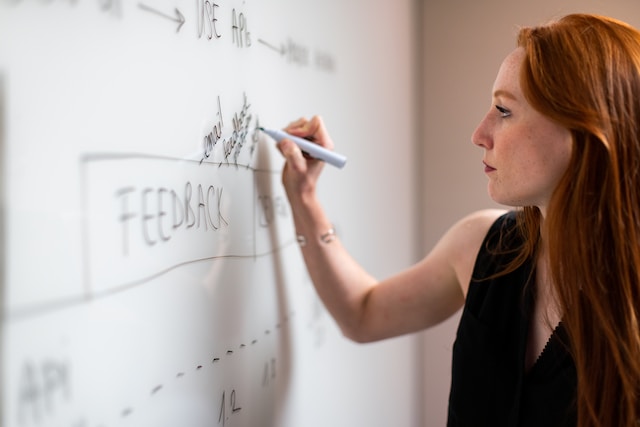
Table of contents
The fields of Artificial Intelligence (AI), Machine Learning (ML), and Big Data have been gaining momentum in recent years, and with that, the demand for professionals in these fields has also increased. Two of the most sought-after roles in these fields are Research Engineer and Deep Learning Engineer. While both roles are related to AI and ML, they differ in their responsibilities, skills, educational backgrounds, tools and software used, common industries, and outlooks. In this article, we will delve into a comprehensive comparison of these two roles.
Definitions
A Research Engineer is a professional who works in the field of research and development. They are responsible for conducting research, developing new technologies, and improving existing ones. They work in a variety of industries, including healthcare, Finance, and technology. Their primary goal is to create innovative solutions to complex problems.
A Deep Learning Engineer is a professional who specializes in the development and implementation of deep learning algorithms. They are responsible for designing, building, and testing deep neural networks to solve complex problems in various industries. They work in a variety of industries, including healthcare, finance, and technology. Their primary goal is to create intelligent systems that can learn and adapt to new data.
Responsibilities
The responsibilities of a Research Engineer and a Deep Learning Engineer differ significantly. A Research Engineer is responsible for conducting research and developing new technologies. They work on projects that are often long-term and require a significant amount of time and resources. They are responsible for identifying problems, developing solutions, and testing their theories. They may also be responsible for writing research papers and presenting their findings to the scientific community.
On the other hand, a Deep Learning Engineer is responsible for designing, building, and testing deep neural networks. They work on projects that are often short-term and require a significant amount of coding and programming. They are responsible for selecting the appropriate algorithm, designing the network Architecture, and tuning the hyperparameters. They may also be responsible for integrating the deep learning model into a larger system.
Required Skills
The skills required for a Research Engineer and a Deep Learning Engineer also differ significantly. A Research Engineer must have strong analytical and problem-solving skills. They must be able to think critically and creatively to develop innovative solutions to complex problems. They must also have strong communication skills to present their findings to the scientific community.
A Deep Learning Engineer must have strong programming skills. They must be proficient in programming languages such as Python, Java, and C++. They must also have a strong understanding of Machine Learning algorithms and deep neural networks. They must be able to design and implement complex algorithms and be able to optimize them for performance.
Educational Background
The educational background required for a Research Engineer and a Deep Learning Engineer also differs. A Research Engineer typically has a Ph.D. in a related field such as Computer Science, mathematics, or physics. They have a strong academic background and have conducted research in their field.
A Deep Learning Engineer typically has a bachelor's or master's degree in computer science, Mathematics, or a related field. They have a strong background in programming and machine learning. They may also have completed additional courses or certifications in deep learning.
Tools and Software Used
The tools and software used by a Research Engineer and a Deep Learning Engineer also differ. A Research Engineer may use a variety of tools and software depending on their field of research. They may use software such as MATLAB, R, or Python for Data analysis and modeling. They may also use simulation software for testing their theories.
A Deep Learning Engineer typically uses tools and software such as TensorFlow, PyTorch, and Keras for building and testing deep neural networks. They may also use cloud-based platforms such as Amazon Web Services (AWS) or Google Cloud Platform (GCP) for training and deploying models.
Common Industries
Research Engineers and Deep Learning Engineers work in a variety of industries. Research Engineers may work in industries such as healthcare, finance, or technology. They may work for academic institutions, government agencies, or private companies.
Deep Learning Engineers may also work in industries such as healthcare, finance, or technology. They may work for companies that specialize in AI and ML, such as Google, Amazon, or Microsoft. They may also work for startups that are developing new AI and ML technologies.
Outlook
The outlook for Research Engineers and Deep Learning Engineers is positive. The demand for professionals in these fields is expected to increase in the coming years. According to the Bureau of Labor Statistics, employment in computer and information technology occupations is projected to grow 11 percent from 2019 to 2029, much faster than the average for all occupations.
Practical Tips for Getting Started
If you are interested in becoming a Research Engineer or a Deep Learning Engineer, here are some practical tips to get started:
- Pursue a degree in a related field such as computer science, mathematics, or Physics.
- Gain experience through internships or research projects.
- Learn programming languages such as Python, Java, and C++.
- Take courses or certifications in machine learning and deep learning.
- Build a portfolio of projects to showcase your skills.
In conclusion, both Research Engineers and Deep Learning Engineers play critical roles in the field of AI and ML. While their responsibilities, skills, educational backgrounds, tools and software used, and common industries differ, both roles offer exciting and rewarding career opportunities. With the increasing demand for professionals in these fields, pursuing a career in research or deep learning can be a smart choice for anyone interested in the intersection of technology and innovation.
AI Research Scientist
@ Vara | Berlin, Germany and Remote
Full Time Senior-level / Expert EUR 70K - 90KData Architect
@ University of Texas at Austin | Austin, TX
Full Time Mid-level / Intermediate USD 120K - 138KData ETL Engineer
@ University of Texas at Austin | Austin, TX
Full Time Mid-level / Intermediate USD 110K - 125KLead GNSS Data Scientist
@ Lurra Systems | Melbourne
Full Time Part Time Mid-level / Intermediate USD 70K - 120KSenior Machine Learning Engineer (MLOps)
@ Promaton | Remote, Europe
Full Time Senior-level / Expert EUR 70K - 110KData Engineering Leader (Facebook Verticals)
@ Meta | Menlo Park, CA | Seattle, WA
Full Time Senior-level / Expert USD 206K - 281K