Deep Learning Engineer vs. Business Data Analyst
The Battle of the Data Titans: Deep Learning Engineer vs Business Data Analyst
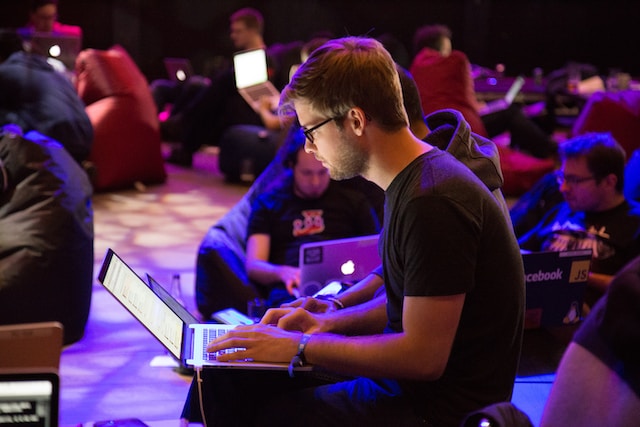
Table of contents
Data is the new oil in the 21st century. The world is generating an enormous amount of data every day, and businesses are trying to extract meaningful insights from it. This has led to the emergence of two hot careers in the data space โ Deep Learning Engineer and Business Data Analyst. In this article, we will compare and contrast these two roles in terms of their definitions, responsibilities, required skills, educational backgrounds, tools and software used, common industries, outlooks, and practical tips for getting started in these careers.
Definitions
A Deep Learning Engineer is a specialist in the field of Artificial Intelligence (AI) and Machine Learning (ML). They design, develop, and implement algorithms and models that can learn from data and make predictions or decisions. They work on complex projects that involve large datasets and require a high level of mathematical and programming skills.
On the other hand, a Business Data Analyst is a professional who uses Data analysis tools and techniques to provide insights into business operations. They collect, analyze, and interpret data to identify trends, patterns, and opportunities for improvement. They work with stakeholders across the organization to provide data-driven insights that inform decision-making.
Responsibilities
The responsibilities of a Deep Learning Engineer include:
- Designing and developing ML models and algorithms
- Collecting, cleaning, and preprocessing data
- Evaluating model performance and making improvements
- Optimizing models for performance and scalability
- Collaborating with cross-functional teams to integrate ML models into products or services
The responsibilities of a Business Data Analyst include:
- Collecting, analyzing, and interpreting data
- Creating reports and visualizations to communicate insights
- Identifying trends, patterns, and opportunities for improvement
- Collaborating with stakeholders to provide data-driven insights that inform decision-making
- Monitoring and evaluating business performance metrics
Required Skills
A Deep Learning Engineer requires the following skills:
- Strong mathematical and statistical skills
- Proficiency in programming languages such as Python, R, or Java
- Knowledge of ML frameworks such as TensorFlow, Keras, or PyTorch
- Experience with data preprocessing and cleaning
- Familiarity with cloud computing platforms such as AWS or Azure
A Business Data Analyst requires the following skills:
- Strong analytical and problem-solving skills
- Proficiency in data analysis tools such as Excel, SQL, or Tableau
- Knowledge of statistical analysis techniques
- Excellent communication and collaboration skills
- Familiarity with business operations and processes
Educational Backgrounds
A Deep Learning Engineer typically requires a Bachelor's or Master's degree in Computer Science, Mathematics, or a related field. They may also have a background in Physics, Engineering, or Statistics. A Ph.D. is not required but can be an advantage.
A Business Data Analyst typically requires a Bachelor's degree in Business Administration, Economics, Mathematics, or Statistics. They may also have a background in Computer Science or Information Systems. A Master's degree is not required but can be an advantage.
Tools and Software Used
A Deep Learning Engineer uses a variety of tools and software, including:
- Programming languages such as Python, R, or Java
- ML frameworks such as TensorFlow, Keras, or PyTorch
- Cloud computing platforms such as AWS or Azure
- Data preprocessing and cleaning tools such as Pandas or NumPy
A Business Data Analyst uses a variety of tools and software, including:
- Data analysis tools such as Excel, SQL, or Tableau
- Statistical analysis software such as R or SAS
- Business Intelligence tools such as Power BI or QlikView
- Data visualization tools such as D3.js or ggplot2
Common Industries
A Deep Learning Engineer can work in a variety of industries, including:
- Healthcare: developing ML models for disease diagnosis or Drug discovery
- Finance: developing ML models for fraud detection or risk management
- Retail: developing ML models for demand forecasting or personalized recommendations
- Automotive: developing ML models for Autonomous Driving or predictive maintenance
A Business Data Analyst can work in a variety of industries, including:
- Finance: analyzing financial data to identify trends or opportunities
- Marketing: analyzing customer data to inform marketing campaigns
- Healthcare: analyzing patient data to improve healthcare outcomes
- Retail: analyzing sales data to optimize inventory management
Outlooks
The outlook for both Deep Learning Engineers and Business Data Analysts is excellent. According to the Bureau of Labor Statistics, employment of computer and information Research scientists (which includes Deep Learning Engineers) is projected to grow 15 percent from 2019 to 2029, much faster than the average for all occupations. Employment of management analysts (which includes Business Data Analysts) is projected to grow 11 percent from 2019 to 2029, much faster than the average for all occupations.
Practical Tips for Getting Started
If you are interested in becoming a Deep Learning Engineer, here are some practical tips:
- Learn programming languages such as Python, R, or Java
- Learn ML frameworks such as TensorFlow, Keras, or PyTorch
- Take online courses or attend boot camps to develop your skills
- Build your own ML projects and contribute to open-source projects
- Network with other ML professionals and attend industry events
If you are interested in becoming a Business Data Analyst, here are some practical tips:
- Learn data analysis tools such as Excel, SQL, or Tableau
- Learn statistical analysis techniques
- Take online courses or attend boot camps to develop your skills
- Build your own data projects and contribute to open-source projects
- Network with other data professionals and attend industry events
Conclusion
In conclusion, both Deep Learning Engineers and Business Data Analysts are in high demand in today's data-driven world. Both roles require different skill sets, educational backgrounds, and tools and software, but they share the same goal of extracting insights from data. Whether you choose to become a Deep Learning Engineer or a Business Data Analyst, the key to success is to keep learning and developing your skills to stay ahead of the curve.
Artificial Intelligence โ Bioinformatic Expert
@ University of Texas Medical Branch | Galveston, TX
Full Time Senior-level / Expert USD 11111111K - 21111111KLead Developer (AI)
@ Cere Network | San Francisco, US
Full Time Senior-level / Expert USD 120K - 160KResearch Engineer
@ Allora Labs | Remote
Full Time Senior-level / Expert USD 160K - 180KEcosystem Manager
@ Allora Labs | Remote
Full Time Senior-level / Expert USD 100K - 120KFounding AI Engineer, Agents
@ Occam AI | New York
Full Time Senior-level / Expert USD 100K - 180KAI Engineer Intern, Agents
@ Occam AI | US
Internship Entry-level / Junior USD 60K - 96K