Data Science Engineer vs. Data Quality Analyst
A Comprehensive Comparison between Data Science Engineer and Data Quality Analyst Roles
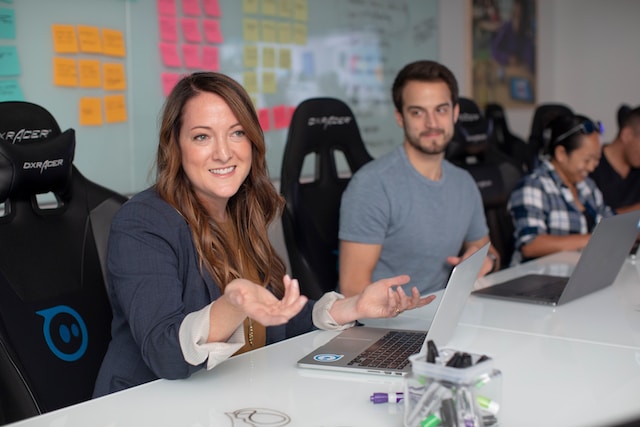
Table of contents
Data Science and Big Data are rapidly growing fields that have become essential for businesses to stay competitive in today's market. With the increasing demand for data-driven insights, the roles of Data Science Engineer and Data Quality Analyst have become crucial for organizations. In this article, we will compare these two roles in detail, including their definitions, responsibilities, required skills, educational backgrounds, tools and software used, common industries, outlooks, and practical tips for getting started in these careers.
Definitions
Data Science Engineer
A Data Science Engineer is responsible for designing, building, and maintaining the infrastructure required for data science projects. They work closely with Data Scientists to develop models and algorithms that can extract insights from data. Data Science Engineers are also responsible for deploying these models and algorithms into production systems.
Data Quality Analyst
A Data quality Analyst is responsible for ensuring that data is accurate, complete, and consistent. They work closely with Data Engineers and Data Scientists to identify data quality issues and develop solutions to address them. Data Quality Analysts also develop and implement data quality standards and policies to ensure that data is of high quality.
Responsibilities
Data Science Engineer
The responsibilities of a Data Science Engineer include:
- Designing and building Data pipelines to collect, process, and store data
- Developing and deploying Machine Learning models and algorithms
- Scaling and optimizing data infrastructure to handle large volumes of data
- Collaborating with Data Scientists to develop and test models and algorithms
- Ensuring that data is stored securely and in compliance with regulatory requirements
Data Quality Analyst
The responsibilities of a Data Quality Analyst include:
- Identifying data quality issues and developing solutions to address them
- Developing and implementing data quality standards and policies
- Collaborating with Data Engineers and Data Scientists to ensure data accuracy and consistency
- Conducting data audits to assess data quality
- Developing and maintaining data quality reports and dashboards
Required Skills
Data Science Engineer
The required skills for a Data Science Engineer include:
- Strong programming skills in languages such as Python, Java, or Scala
- Experience with data processing frameworks such as Hadoop, Spark, or Flink
- Experience with machine learning frameworks such as TensorFlow or PyTorch
- Knowledge of database systems such as SQL and NoSQL
- Familiarity with cloud computing platforms such as AWS, Azure, or GCP
Data Quality Analyst
The required skills for a Data Quality Analyst include:
- Strong analytical skills
- Knowledge of data quality standards and best practices
- Experience with data profiling and data cleansing tools
- Knowledge of database systems such as SQL and NoSQL
- Familiarity with Data visualization tools such as Tableau or Power BI
Educational Backgrounds
Data Science Engineer
The educational backgrounds for a Data Science Engineer include:
- Bachelor's or Master's degree in Computer Science, Data Science, or a related field
- Strong programming skills in languages such as Python, Java, or Scala
- Experience with data processing frameworks such as Hadoop, Spark, or Flink
- Knowledge of machine learning algorithms and frameworks
Data Quality Analyst
The educational backgrounds for a Data Quality Analyst include:
- Bachelor's or Master's degree in Computer Science, Data Science, or a related field
- Knowledge of data quality standards and best practices
- Experience with data profiling and data cleansing tools
- Knowledge of database systems such as SQL and NoSQL
Tools and Software Used
Data Science Engineer
The tools and software used by a Data Science Engineer include:
- Programming languages such as Python, Java, or Scala
- Data processing frameworks such as Hadoop, Spark, or Flink
- Machine learning frameworks such as TensorFlow or PyTorch
- Database systems such as SQL and NoSQL
- Cloud computing platforms such as AWS, Azure, or GCP
Data Quality Analyst
The tools and software used by a Data Quality Analyst include:
- Data profiling and data cleansing tools such as Talend or Informatica
- Database systems such as SQL and NoSQL
- Data visualization tools such as Tableau or Power BI
Common Industries
Data Science Engineer
The common industries that employ Data Science Engineers include:
- Technology
- Finance
- Healthcare
- Retail
- Manufacturing
Data Quality Analyst
The common industries that employ Data Quality Analysts include:
- Finance
- Healthcare
- Retail
- Manufacturing
- Government
Outlooks
Data Science Engineer
The outlook for Data Science Engineers is excellent, with a projected job growth rate of 15% from 2019 to 2029. The demand for data-driven insights is increasing in all industries, and Data Science Engineers are essential for developing and deploying machine learning models and algorithms.
Data Quality Analyst
The outlook for Data Quality Analysts is also excellent, with a projected job growth rate of 11% from 2019 to 2029. As organizations continue to rely on data to make decisions, the need for high-quality data will only increase. Data Quality Analysts will be essential for ensuring that data is accurate, complete, and consistent.
Practical Tips for Getting Started
Data Science Engineer
If you want to become a Data Science Engineer, here are some practical tips:
- Learn programming languages such as Python, Java, or Scala
- Gain experience with data processing frameworks such as Hadoop, Spark, or Flink
- Learn machine learning algorithms and frameworks such as TensorFlow or PyTorch
- Build a portfolio of projects that demonstrate your skills
- Consider earning a Master's degree in Computer Science, Data Science, or a related field
Data Quality Analyst
If you want to become a Data Quality Analyst, here are some practical tips:
- Learn data quality standards and best practices
- Gain experience with data profiling and data cleansing tools such as Talend or Informatica
- Learn database systems such as SQL and NoSQL
- Build a portfolio of projects that demonstrate your skills
- Consider earning a Master's degree in Computer Science, Data Science, or a related field
Conclusion
In conclusion, both Data Science Engineers and Data Quality Analysts are essential for organizations that rely on data to make decisions. While their roles may differ, they both require strong technical skills and a deep understanding of data. By understanding the differences between these roles, you can choose the career path that best suits your interests and skills.
Lead Developer (AI)
@ Cere Network | San Francisco, US
Full Time Senior-level / Expert USD 120K - 160KResearch Engineer
@ Allora Labs | Remote
Full Time Senior-level / Expert USD 160K - 180KEcosystem Manager
@ Allora Labs | Remote
Full Time Senior-level / Expert USD 100K - 120KFounding AI Engineer, Agents
@ Occam AI | New York
Full Time Senior-level / Expert USD 100K - 180KAI Engineer Intern, Agents
@ Occam AI | US
Internship Entry-level / Junior USD 60K - 96KAI Research Scientist
@ Vara | Berlin, Germany and Remote
Full Time Senior-level / Expert EUR 70K - 90K