Applied Scientist vs. Data Quality Analyst
A Comprehensive Comparison between Applied Scientist and Data Quality Analyst Roles
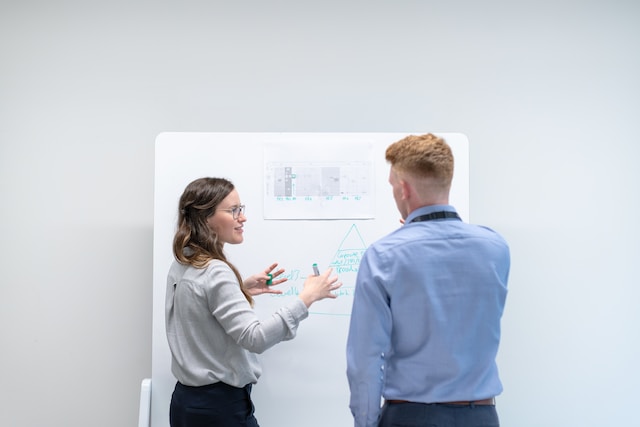
Table of contents
In today's digital age, the fields of Artificial Intelligence (AI), Machine Learning (ML), and Big Data are becoming increasingly popular. As a result, there is a growing demand for professionals with expertise in these areas. Two such roles are Applied Scientist and Data quality Analyst. In this article, we will provide a detailed comparison between these two roles, covering their definitions, responsibilities, required skills, educational backgrounds, tools and software used, common industries, outlooks, and practical tips for getting started in these careers.
Definitions
An Applied Scientist is a professional who applies scientific principles to solve real-world problems. They work on developing and implementing machine learning models, algorithms, and statistical models to solve complex problems in various domains, such as healthcare, Finance, and E-commerce. Applied Scientists are responsible for designing, developing, and deploying ML models that can improve the efficiency and accuracy of business processes.
On the other hand, a Data quality Analyst is a professional who ensures the accuracy and completeness of data. They are responsible for identifying and resolving data quality issues, such as missing values, incorrect data types, and inconsistencies. Data Quality Analysts work on data profiling, data cleansing, data validation, and data enrichment to ensure that the data is accurate, reliable, and consistent.
Responsibilities
The responsibilities of an Applied Scientist and a Data Quality Analyst are significantly different. An Applied Scientist is responsible for developing and implementing ML models to solve complex business problems. They work on data collection, feature Engineering, model selection, and hyperparameter tuning to ensure that the ML models are accurate and efficient. Applied Scientists also work on integrating ML models into business processes and monitoring their performance.
On the other hand, a Data Quality Analyst is responsible for ensuring the accuracy and completeness of data. They work on data profiling, data cleansing, and data validation to ensure that the data is accurate and reliable. Data Quality Analysts also work on data enrichment to improve the quality of data. They collaborate with data engineers, data scientists, and business analysts to ensure that the data is accurate and consistent.
Required Skills
The required skills for an Applied Scientist and a Data Quality Analyst are significantly different. An Applied Scientist needs to have a strong background in Statistics, Mathematics, and Computer Science. They should have expertise in machine learning algorithms, data structures, and programming languages such as Python, R, and Java. Applied Scientists should also have excellent problem-solving skills and the ability to work with large datasets.
On the other hand, a Data Quality Analyst needs to have a strong background in Data management, Data analysis, and data quality. They should have expertise in data profiling, data cleansing, and data validation. Data Quality Analysts should also have excellent analytical and problem-solving skills and the ability to work with different data management tools and software.
Educational Backgrounds
The educational backgrounds of an Applied Scientist and a Data Quality Analyst are also different. An Applied Scientist typically has a Master's or Ph.D. degree in Computer Science, Mathematics, or Statistics. They may also have a background in Physics, Engineering, or other related fields. Applied Scientists should have expertise in machine learning, statistics, and programming.
On the other hand, a Data Quality Analyst typically has a Bachelor's or Master's degree in Computer Science, Information Science, or a related field. They may also have a background in Business Administration, Economics, or other related fields. Data Quality Analysts should have expertise in data management, data analysis, and data quality.
Tools and Software Used
The tools and software used by an Applied Scientist and a Data Quality Analyst are different. An Applied Scientist typically uses tools and software such as Python, R, TensorFlow, PyTorch, and Scikit-learn for developing and implementing ML models. They may also use cloud platforms such as AWS, Azure, or Google Cloud for deploying ML models.
On the other hand, a Data Quality Analyst typically uses tools and software such as SQL, Excel, Talend, Informatica, and Alteryx for data profiling, data cleansing, and data validation. They may also use data quality tools such as Trillium, IBM InfoSphere, or SAP Information Steward for ensuring data quality.
Common Industries
The industries in which an Applied Scientist and a Data Quality Analyst work are also different. An Applied Scientist typically works in industries such as healthcare, finance, e-commerce, and transportation. They may also work in Research and development labs, academic institutions, or government agencies.
On the other hand, a Data Quality Analyst typically works in industries such as Banking, insurance, healthcare, and retail. They may also work in data management companies, Consulting firms, or government agencies.
Outlooks
The outlooks for an Applied Scientist and a Data Quality Analyst are positive. According to the Bureau of Labor Statistics, the employment of computer and information Research scientists, which includes Applied Scientists, is projected to grow 15 percent from 2019 to 2029, much faster than the average for all occupations. On the other hand, the employment of computer and information systems managers, which includes Data Quality Analysts, is projected to grow 10 percent from 2019 to 2029, much faster than the average for all occupations.
Practical Tips for Getting Started
If you are interested in pursuing a career as an Applied Scientist, you should focus on developing your skills in machine learning, statistics, and programming. You should also gain experience in working with large datasets and deploying ML models on cloud platforms. You may also consider obtaining a Master's or Ph.D. degree in Computer Science, Mathematics, or Statistics.
On the other hand, if you are interested in pursuing a career as a Data Quality Analyst, you should focus on developing your skills in data management, Data analysis, and data quality. You should also gain experience in working with different data management tools and software. You may also consider obtaining a Bachelor's or Master's degree in Computer Science, Information Science, or a related field.
In conclusion, both Applied Scientist and Data Quality Analyst roles are essential for organizations that deal with large amounts of data. While the roles have different responsibilities, required skills, and educational backgrounds, they both offer promising career paths in the AI/ML and Big Data space.
Data Architect
@ University of Texas at Austin | Austin, TX
Full Time Mid-level / Intermediate USD 120K - 138KData ETL Engineer
@ University of Texas at Austin | Austin, TX
Full Time Mid-level / Intermediate USD 110K - 125KLead GNSS Data Scientist
@ Lurra Systems | Melbourne
Full Time Part Time Mid-level / Intermediate USD 70K - 120KSenior Machine Learning Engineer (MLOps)
@ Promaton | Remote, Europe
Full Time Senior-level / Expert EUR 70K - 110KSenior Data Quality and Governance Analyst
@ JLL | Home Office - Tennessee
Full Time Senior-level / Expert USD 70KSoftware Engineering Manager, PyTorch Compiler
@ Meta | Menlo Park, CA
Full Time Mid-level / Intermediate USD 177K - 251K